Central limit theorem describes the behavior of the sampling distribution of the mean of a random variable,
It allows to make inferences about populations based on samples, distributions of sample means approaches normal distributions, even when the original experiment doesn’t follow a normal distribution
For example,
Let’s imagine we calculate mean of the sample, 5 times/trials(n=5) and plot mean of sample on the graph. As the sample size increases say n=1000, the sample mean plot almost looks normal distribution (tend to form a bell curve centered around the population mean) This distribution is called as Sample mean distribution
Central limit theorem, also gives us the mean and standard deviation of the Sample mean distribution
Standard deviation of sampling distribution (σ_mean) = σ / √n
Mean: The sampling distribution of the means will have a mean equal to the mean (μ) of the original population (because of normal distribution)
Standard deviation (σ_mean) equal to the standard deviation of the original population (σ) divided by the square root of the sample size (n)
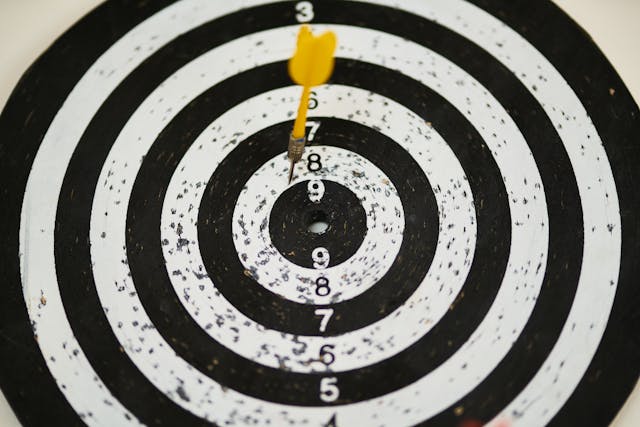